DBpedia Member Features – Over the last year we gave DBpedia members multiple chances to present their work, tools and applications. In this way, our members gave exclusive insights on the DBpedia blog. This time we will continue with Diffbot, a California-based company whose mission is to “extract knowledge in an automated way from documents.” They will introduce the Diffbot Knowledge Graph and present topics, like Market Intelligence and Ecommerce. Have fun reading!
by Filipe Mesquita & Merrill Cook, Diffbot
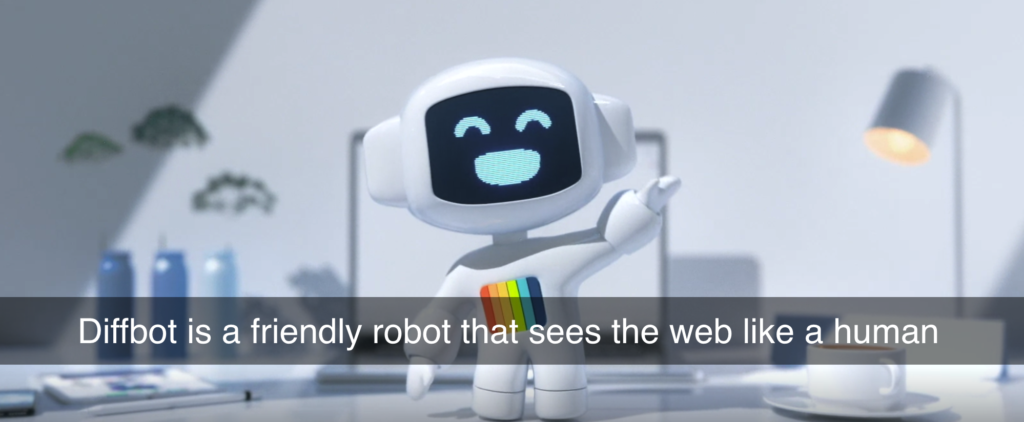
Diffbot is on a mission to create a knowledge graph of the entire public web. We are teaching a robot, affectionately known as Diffy, to read the web like a human and translate its contents into a format that (other perhaps less sophisticated) machines can understand. All of this information is linked and cleaned on a continuous basis to populate the Diffbot Knowledge Graph.
The Diffbot Knowledge Graph already contains billions of entities, including over 240M organizations, 700M people, 140M products, and 1.6B news articles. This scale is only possible because Diffy is fully autonomous and doesn’t depend on humans to build the Diffbot Knowledge Graph. Using cutting-edge crawling technology, natural language processing, and computer vision, Diffy is able to read and extract facts from across the entire web.
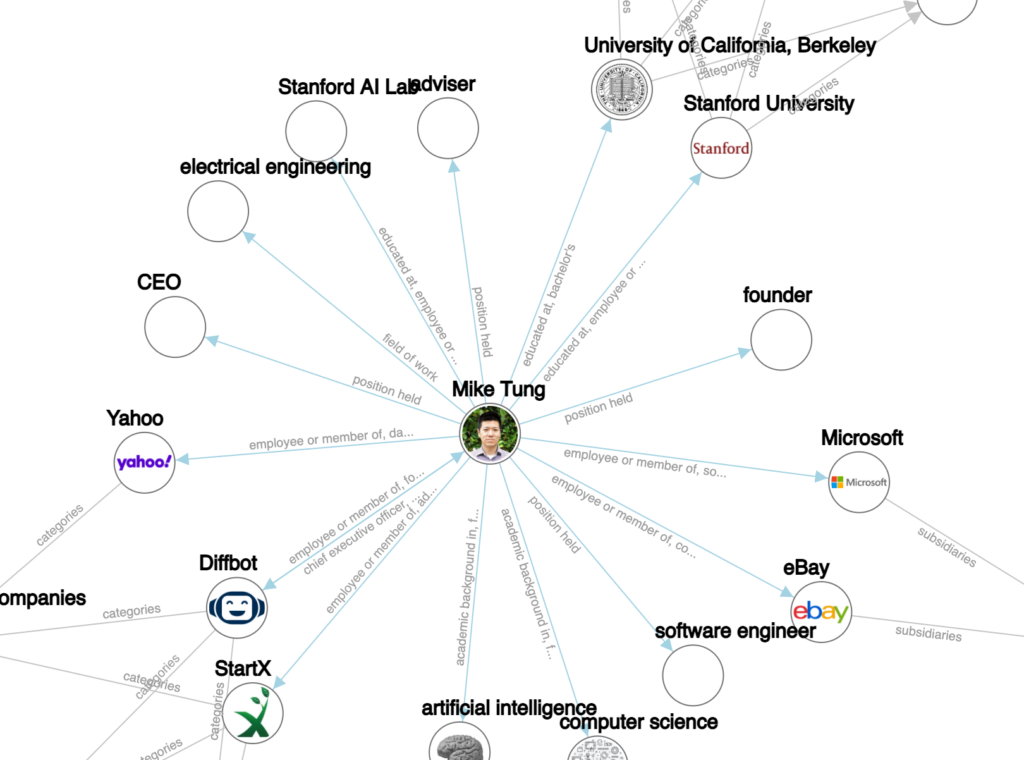
While we believe a knowledge graph like Diffbot’s will be used by virtually every organization one day, there are 4 use cases where the Diffbot Knowledge Graph excels today: (1) Market Intelligence, (2) News Monitoring, (3) E-commerce, and (4) Machine learning.
Market Intelligence
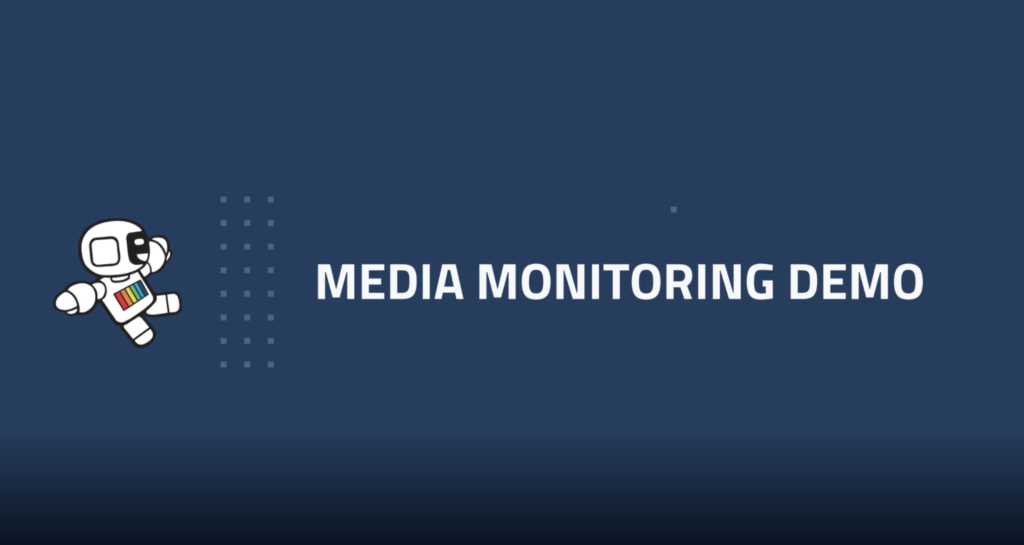
Video: https://www.diffbot.com/assets/video/solutions-for-media-monitoring.mp4
At its simplest, market intelligence is the generation of insights about participants in a market. These can include customers, suppliers, competitors, as well as attitudes of the general public and political establishment.
While market intelligence data is all over the public web, this can be a “double-edged sword.” The range of potential sources for market intelligence data can exhaust the resources of even large teams performing manual fact accumulation.
Diffbot’s automated web data extraction eliminates the inefficiencies of manual fact gathering. Without such automation, it’s simply not possible to monitor everything about a company across the web.
We see market intelligence as one of the most well-developed use cases for the Diffbot Knowledge Graph. Here’s why:
- The Diffbot Knowledge Graph is built around organizations, people, news articles, products, and the relationships among them. These are the types of facts that matter in market intelligence.
- Knowledge graphs have flexible schemas, allowing for new fact types to be added “on the fly” as the things we care about in the world change
- Knowledge graphs provide unique identifiers for all entities, supporting the disambiguation entities like Apple (the company) vs apple (the fruit).
Market intelligence uses from our customers include:
- Querying the Knowledge Graph for companies that fit certain criteria (size, revenue, industry, location) rather than manually searching for them in Google
- Creating dashboards to receive insights about companies in a certain industry
- Improving an internal database by using the data from the Diffbot Knowledge Graph.
- Custom solutions that incorporate multiple Diffbot products (custom web crawling, natural language processing, and Knowledge Graph data consumption)
News Monitoring
Sure, the news is all around us. But most companies are overwhelmed by the sheer amount of information produced every day that can impact their business.
The challenges faced by those trying to perform news monitoring on unstructured article data are numerous. Articles are structured differently across the web, making aggregation of diverse sources difficult. Many sources and aggregators silo their news by geographic location or language.
Strengths of providing article data through a wider Knowledge Graph include the ability to link articles to the entities (people, organizations, locations, etc) mentioned in each article. Additional natural language processing includes the ability to identify quotes and who said them as well as the sentiment of the article author towards each entity mentioned in the article.
In high-velocity, socially-fueled media, the need for automated analysis of information in textual form is even more pressing. Among the many applications of our technology, Diffbot is helping anti-bias and misinformation initiatives with partnerships involving FactMata as well as the European Journalism Centre.
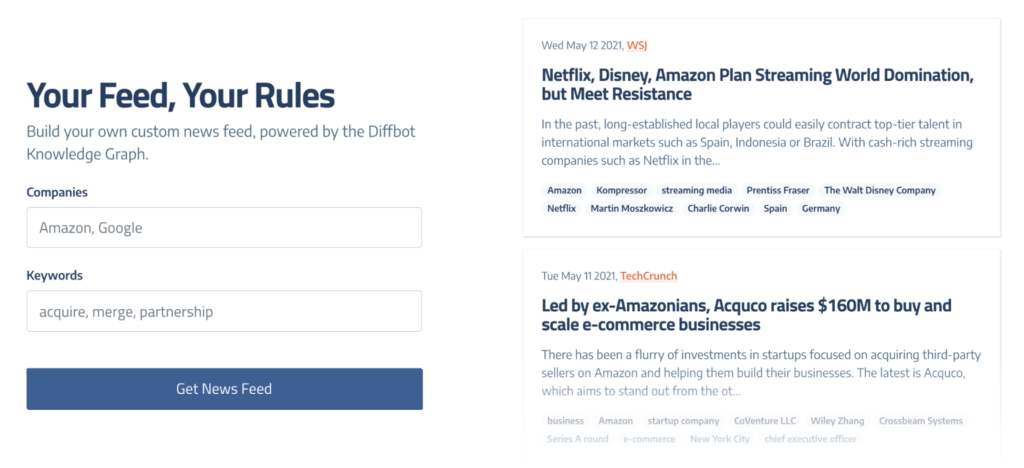
Check out how easy it is to build your own custom pan-lingual news feed in our news feed builder.
Ecommerce
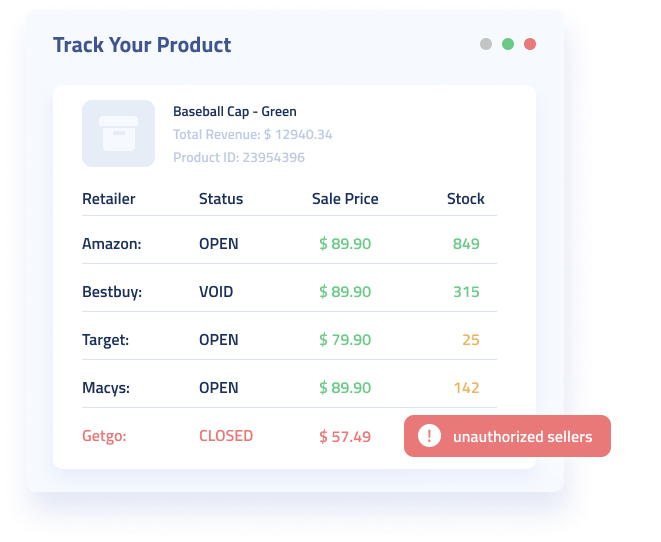
Many of the largest names in ecommerce have utilized Diffbot’s ability to transform unstructured product, review, and discussion data into valuable ecommerce intelligence. Whether pointing AI-enabled crawlers at their own marketplaces to detect fraudulent, duplicate, or underperforming products, or by analyzing competitor or supplier product listings.
One of the benefits of utilizing Diffbot’s AI-enabled product API or our product entities within the Knowledge Graph is the difficulty of scraping product data at scale. Many ecommerce sites employ active measures to make the scraping of their pages at scale difficult. We’ve already built out the infrastructure and can begin returning product data at scale in minutes.
The use of rule-based scraping by many competitors or in-house teams means that whenever ecommerce sites shift their layout or you try to extract ecommerce web data from a new location, your extraction method is likely to break. Additionally, hidden or toggleable fields on many ecommerce pages are more easily extracted by solutions with strong machine vision capabilities.
Diffbot’s decade-long focus on natural language processing also allows the inclusion of rich discussion data parsed for entities, connections, and sentiment. On large ecommerce sites, the structuring and additional processing of review data can be a large feat and provide high value.
Machine Learning
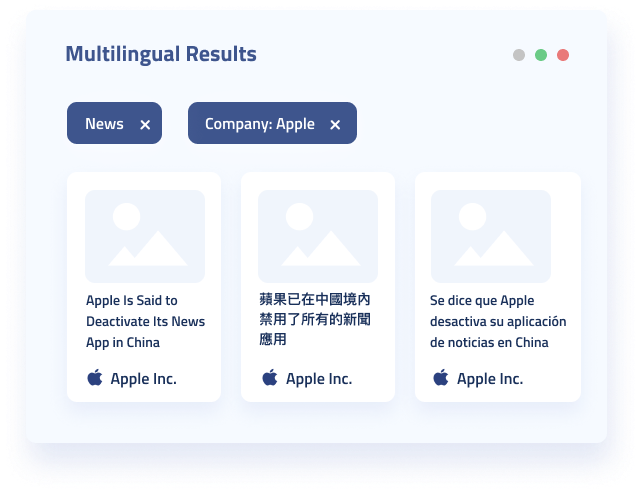
Even when you can get your hands on the right raw data to train machine learning models, cleaning and labeling the data can be a costly process. To help with this, Diffbot’s Knowledge Graph provides potentially the largest selection of once unstructured web data, complete with data provenance and confidence scores for each fact.
Our customers use a wide range of web data to quickly and accurately train models on diverse data types. Need highly informal text input from reviews? Video data in a particular language? Product or firmographic data? It’s all in the Knowledge Graph, structured and with API access so customers can quickly jump into validating new models.
With a long association with Stanford University and many research partnerships, Diffbot’s experts in web-scale machine learning work in tandem with many customers to create custom solutions and mutually beneficial partnerships.
To some, 2020 was the year of the knowledge graph. And while innovative organizations have long seen the benefits of graph databases, recent developments in the speed of fact accumulation online mean the future of graphs has never been more bright.
A big thank you to Diffbot, especially to Filipe Mesquita and Merrill Cook for presenting the Diffbot Knowledge Graph.
Yours,
DBpedia Association
- Did you consider this information as helpful?
- Yep!Not quite ...