DBpedia Member Feature – Over the last year we gave DBpedia members multiple chances to present their work, tools and applications. In this way, our members gave exclusive insights on the DBpedia blog. This time we will continue with Wallscope, who supports both organisational goals, improves existing processes and embed new technologies by generating the insights that power change. David Eccles presents the opportunities of digital audio. Have fun reading!
by David Eccles, Wallscope
Motivation
The use of digital audio has accelerated throughout the pandemic, creating a cultural shift in the use of audio form content within business and consumer communications.
Alongside this the education and entertainment industries embraced semantic technologies as a means to develop sustainable delivery platforms under very difficult circumstances.
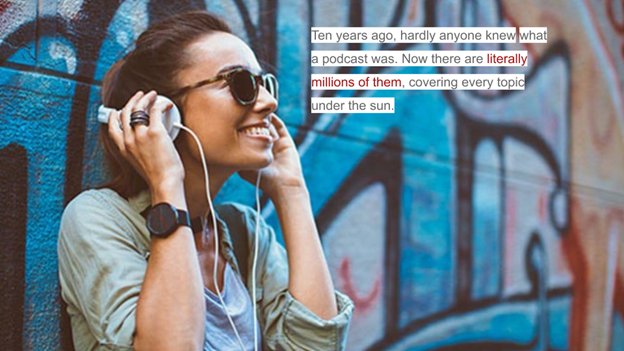
Wallscope’s research and development activities were already aligned to exploring speech-driven applications and through this we engaged with Edinburgh University’s Creative Informatics department to explore practical use cases focusing on enhancing the content of podcasts.
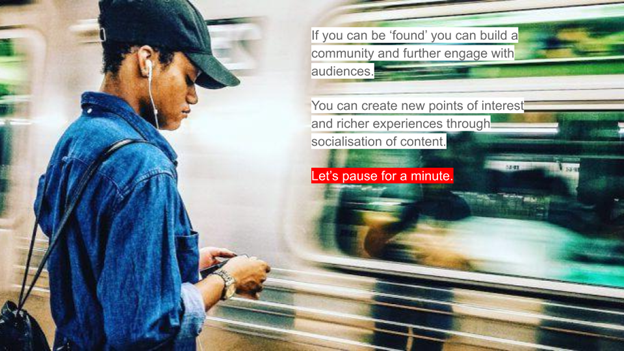
Our focus now is on how user experience can be enhanced with knowledge graph interaction, providing contextually relevant information to add value to the overall experience. As DBpedia provides the largest knowledge repository available, Wallscope embedded semantic queries to the service into the resulting workflow.
Speech to Linked Data
Speech-driven applications require a high level of accuracy and are notoriously difficult to develop, as anyone with experience of spoken dialog systems will probably be aware. A range of Natural Language Processing models are available which perform with a high degree of accuracy – particularly for basic tasks such as Named Entity Recognition – to recognise people, places, and organisations (spaCy and PyTorch are good examples of this). Obviously the tasks become more difficult to achieve when inherently complicated concepts are brought into the mix such as cultural references and emotional reactions.
To this end Wallscope re-deployed and trained a machine learning model called BERT. This stands for Bidirectional Encoder Representations from Transformers and it is a technique for NLP pre-training originally developed by Google.
BERT uses the mechanism of “paying attention” to better understand the contextual relationships between each word (or sub-words) within a sentence. Having previous experience deploying BERT models within the healthcare industry, we adapted and trained the model on a variety of podcast conversations.
As an example of how this works in practice, consider the phrase “It looked like a painting”. BERT looks at the word “it” and then checks its relationship with every other word in the sentence. This way, BERT can tell that “it” refers strongly to “painting”. This allows BERT to understand the context of each word within a given sentence.
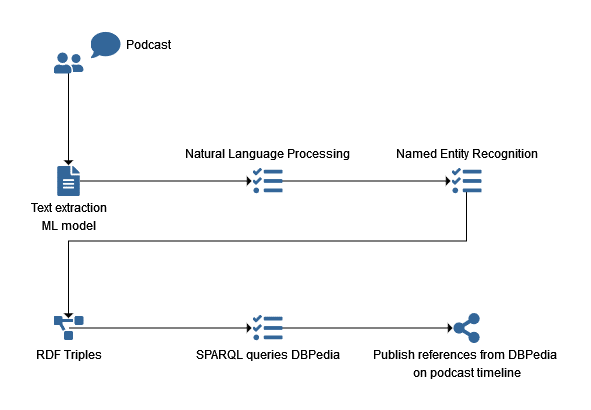
We then looked at how this could be used to better engage users across the podcast listening experience, and provide points of knowledge expansion, engagement and ‘socialisation’ of content in web-based environments. This in turn can create a richer and more meaningful experience for listeners that runs in parallel with podcasting platforms.
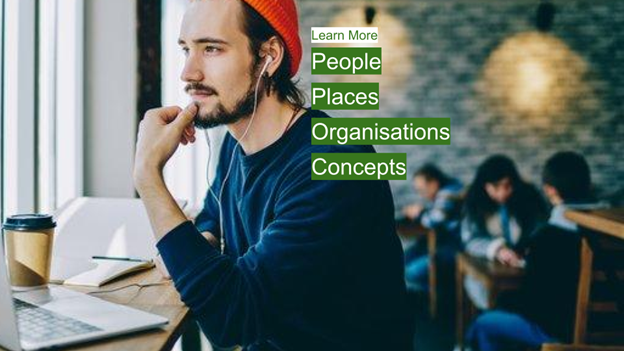
Working across multiple files containing podcast format audio, we looked at several areas of improvements for listeners, creators and researchers. Our primary aim was to demonstrate the value of semantic enhancements to the transcriptions.
We worked with these across several processes to enhance them with Named Entity Recognition using our existing stack. From there we extended the analysis of ‘topics’ using a blend of Machine Learning models. That very quickly allowed us to gain a deep understanding of the relationships contained with the spoken word content. By visualising that we could gain a deeper insight into the content and how it could be better presented, by reconciling it with references within DBpedia.
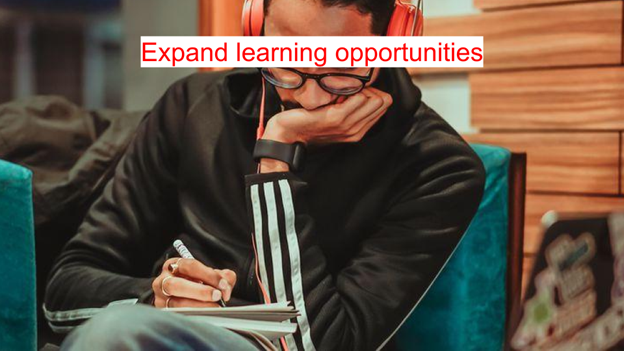
This analysis led us to ideate around an interface that was built around the timeline presented by the audio content.
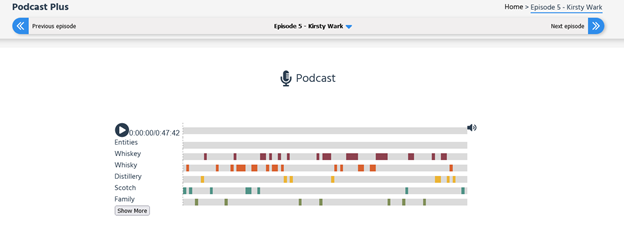
Playback of audio with related terms
This allows the listener to gain contextually related insights by dynamically querying DBpedia for entities extracted from the podcast itself. This knowledge extension is valuable to enhance not only the listeners’ experience but also to provide a layer of ‘stickiness’ for the content across the internet as it enhances findability.
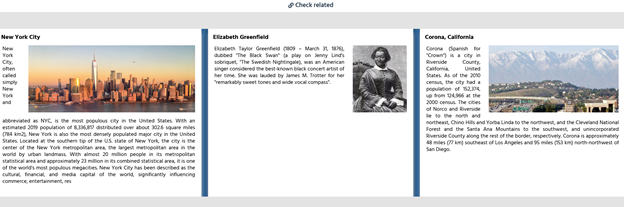
This shows how knowledge can be added to a page using DBpedia.
One challenge is the quality of transcriptions. With digital speech recognition, there is never a 100% confidence level across unique audio recordings such as podcasts as well as within video production.
We are currently working with services which are increasingly harnessing AI technologies to not only improve the quality of transcription but also the insights which can be derived from spoken word data sources. A current area of research for Wallscope is how our ML models can be utilised to improve the curation layer of transcripts. This is important as keeping the human in the loop is critical to ensure the fidelity of any transcription process. By deploying the same techniques – albeit in reverse – there is an interesting opportunity to create dynamic ‘sense-checking’ models. While this is at an early stage, DBpedia undoubtedly will be an important part of that.
We are also developing some visualisation techniques to assist curators to identify ‘errors’ and to provide suggestions for more robust topic classification models. This allows more generalised suggestions for labels. For example while we may have a specific reference to ‘zombie’ to present that as a subset of ‘horror’ has more value in categorisation systems. Another example could relate to location. If we identify ‘France’ in a transcription with 100% certainty, then we can create greater certainty around ‘Paris’ as being Paris, France as opposed to Paris,Texas. This also applies to machine learning-based summarisation techniques.
Next steps
We are further exploring how these approaches can best assist in the exploration of archives as well as incorporating text analysis to improve the actual curation of archives.
Please contact Ian Allaway or David Eccles for more information, or visit www.wallscope.co.uk
Further reading on ‘Podcasting Exploration’
- Did you consider this information as helpful?
- Yep!Not quite ...